Foundations of Probability in Python - Datacamp course | Solution
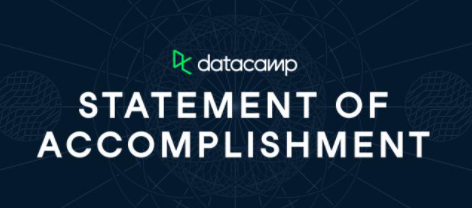
Course Description
Probability is the study of regularities that emerge in the outcomes of random experiments. In this course, you’ll learn about fundamental probability concepts like random variables (starting with the classic coin flip example) and how to calculate mean and variance, probability distributions, and conditional probability. We’ll also explore two very important results in probability: the law of large numbers and the central limit theorem. Since probability is at the core of data science and machine learning, these concepts will help you understand and apply models more robustly. Chances are everywhere, and the study of probability will change the way you see the world. Let’s get random!
Chapter -1
1. Let’s start flipping coins
A coin flip is the classic example of a random experiment. The possible outcomes are heads or tails. This type of experiment, known as a Bernoulli or binomial trial, allows us to study problems with two possible outcomes, like “yes” or “no” and “vote” or “no vote.” This chapter introduces Bernoulli experiments, binomial distributions to model multiple Bernoulli trials, and probability simulations with the scipy library.
Flipping coins
This exercise requires the bernoulli object from the scipy.stats library to simulate the two possible outcomes from a coin flip, 1 (“heads”) or 0 (“tails”), and the numpy library (loaded as np) to set the random generator seed.
You’ll use the bernoulli.rvs() function to simulate coin flips using the size argument.
You will set the random seed so you can reproduce the results for the random experiment in each exercise.
From each experiment, you will get the values of each coin flip. You can add the coin flips to get the number of heads after flipping 10 coins using the sum() function.
1. Import bernoulli from scipy.stats, set the seed with np.random.seed(). Simulate 1 flip, with a 35% chance of heads.
from scipy.stats import bernoulli
np.random.seed(42)
coin_flip = bernoulli.rvs(p=.35, size=1)
print(coin_flip)
Use bernoulli.rvs() and sum() to get the number of heads after 10 coin flips with 35% chance of getting heads.
# Import the bernoulli object from scipy.stats
from scipy.stats import bernoulli
# Set the random seed to reproduce the results
np.random.seed(42)
# Simulate ten coin flips and get the number of heads
ten_coin_flips = bernoulli.rvs(p=.35, size=10)
coin_flips_sum = sum(ten_coin_flips)
print(coin_flips_sum)
Using bernoulli.rvs() and sum(), try to get the number of heads after 5 flips with a 50% chance of getting heads.
# Import the bernoulli object from scipy.stats
from scipy.stats import bernoulli
# Set the random seed to reproduce the results
np.random.seed(42)
# Simulate ten coin flips and get the number of heads
five_coin_flips = bernoulli.rvs(p=.50, size=5)
coin_flips_sum = sum(five_coin_flips)
print(coin_flips_sum)
Using binom to flip even more coins
Previously, you simulated 10 coin flips with a 35% chance of getting heads using bernoulli.rvs().
This exercise loads the binom object from scipy.stats so you can use binom.rvs() to simulate 20 trials of 10 coin flips with a 35% chance of getting heads on each coin flip.
Use the binom.rvs() function to simulate 20 trials of 10 coin flips with a 35% chance of getting heads.
# Set the random seed to reproduce the results
np.random.seed(42)
# Simulate 20 trials of 10 coin flips
draws = binom.rvs(n=10, p=.35, size=20)
print(draws)
Predicting the probability of defects
Any situation with exactly two possible outcomes can be modeled with binomial random variables. For example, you could model if someone likes or dislikes a product, or if they voted or not.
Let’s model whether or not a component from a supplier comes with a defect. From the thousands of components that we got from a supplier, we are going to take a sample of 50, selected randomly. The agreed and accepted defect rate is 2%.
We import the binom object from scipy.stats.
Recall that:
binom.pmf() calculates the probability of having exactly k heads out of n coin flips.
binom.cdf() calculates the probability of having k heads or less out of n coin flips.
binom.sf() calculates the probability of having more than k heads out of n coin flips.
Question
Let’s answer a simple question before we start calculating probabilities:
What is the probability of getting more than 20 heads from a fair coin after 30 coin flips?
Possible Answers
· binom.pmf(k=20, n=30, p=0.5)
· 1 — binom.pmf(k=20, n=30, p=0.5)
· binom.sf(k=20, n=30, p=0.5) — Answer
· binom.cdf(k=20, n=30, p=0.5)
# Probability of getting exactly 1 defective component
prob_one_defect = binom.pmf(k=1, n=50, p=0.02)
print(prob_one_defect)
# Probability of not getting any defective components
prob_no_defects = binom.pmf(k=0, n=50, p=0.02)
print(prob_no_defects)
# Probability of getting 2 or less defective components
prob_two_or_less_defects = binom.cdf(k=2, n=50, p=0.02)
print(prob_two_or_less_defects)
Predicting employment status
Consider a survey about employment that contains the question “Are you employed?” It is known that 65% of respondents will answer “yes.” Eight survey responses have been collected.
We load the binom object from scipy.stats with the following code: from scipy.stats import binom
Answer the following questions using pmf(), cdf(), and sf().
Calculate the probability of getting exactly 5 yes responses.
# Calculate the probability of getting exactly 5 yes responses
prob_five_yes = binom.pmf(k=5, n=8, p=0.65)
print(prob_five_yes)
Calculate the probability of getting 3 or fewer no responses.
prob_three_or_less_no = 1-binom.cdf(k=3, n=8, p=0.65)
print(prob_three_or_less_no)
Calculate the probability of getting more than 3 yes responses using binom.sf().
prob_more_than_three_yes = binom.sf(k=3, n=8, p=0.65)
print(prob_more_than_three_yes)
Predicting burglary conviction rate
four_solved = binom.pmf(k=4, n=9, p=0.20)
print(four_solved)
more_than_three_solved = binom.sf(k=3, n=9, p=0.2)
print(more_than_three_solved)
two_or_three_solved = binom.pmf(k=2, n=9, p=0.2) + binom.pmf(k=3, n=9, p=0.2)
print(two_or_three_solved)
tail_probabilities = binom.cdf(k=1, n=9, p=0.2) + binom.sf(k=7, n=9, p=0.2)
print(tail_probabilities)
How do you calculate the expected value and the variance from a binomial distribution with parameters n=10 and p=0.25?
Possible Answers
binom.stats(n=10, p=0.25) — Ans
binom.pmf(k=5, n=10,p=0.25)
describe(binom.rvs(n=10, p=0.25, size=100)).mean
describe(binom.rvs(n=10, p=0.25, size=100)).variance
Calculating the sample mean
sample_of_100_flips = binom.rvs(n=1, p=0.5, size=100)
sample_mean_100_flips = describe(sample_of_100_flips).mean
print(sample_mean_100_flips)
sample_mean_1000_flips = describe(binom.rvs(n=1, p=0.5, size=1000)).mean
sample_mean_2000_flips = describe(binom.rvs(n=1, p=0.5, size=2000)).mean
print(sample_mean_2000_flips)
Checking the result
sample = binom.rvs(n=10, p=0.3, size=2000)
sample_describe = describe(sample)
mean = 10*0.3
variance = mean*(1–0.3)
binom_stats = binom.stats(n=10, p=0.3)
print(sample_describe.mean, sample_describe.variance, mean, variance, binom_stats)
Calculating the mean and variance of a sample
for i in range(0, 1500):
sample = binom.rvs(n=10, p=0.25, size=10)
averages.append(describe(sample).mean)
variances.append(describe(sample).variance)
print(“Mean {}”.format(describe(averages).mean))
print(“Variance {}”.format(describe(variances).mean))
print(binom.stats(n=10, p=0.25))
Chepter -2
Any overlap?
When you calculate probabilities for multiple events the most important thing to notice is the relation between the events, in particular, if there is any overlap between any two events.
· What would be the formula to calculate the probability of A or B, given that A and B are not mutually exclusive?
Possible Answers
· P(A and B) = P(A) + P(B)
· P(A or B) = P(A) — P(B) + P(A and B)
· P(A or B) = P(A)P(B) — P(A and B)
· P(A or B) = P(A) + P(B) — P(A and B) — Ans
Measuring a sample
# Count how many times you got 2 heads from the sample data
count_2_heads = find_repeats(sample_of_two_coin_flips).counts[2]
# Divide the number of heads by the total number of draws
prob_2_heads = count_2_heads / 1000
# Display the result
print(prob_2_heads)
# Get the relative frequency from sample_of_two_coin_flips
# Set numbins as 3
# Extract frequency
rel_freq = relfreq(sample_of_two_coin_flips, numbins=3).frequency
print(rel_freq)
# Probability of getting 0, 1, or 2 from the distribution
probabilities = binom.pmf([0,1,2], n=2, p=0.5)
print(probabilities)
Joint probabilities
# Individual probabilities
P_Eng_works = 0.99
P_GearB_works = 0.995
# Joint probability calculation
P_both_works = P_Eng_works * P_GearB_works
print(P_both_works)
# Individual probabilities
P_Eng_fails = 0.01
P_Eng_works = 0.99
P_GearB_fails = 0.005
P_GearB_works = 0.995
# Joint probability calculation
P_only_GearB_fails = P_GearB_fails * P_Eng_works
P_only_Eng_fails = P_Eng_fails * P_GearB_works
# Calculate result
P_one_fails = P_only_GearB_fails + P_only_Eng_fails
print(P_one_fails)
# Individual probabilities
P_Eng_fails = 0.01
P_Eng_works = 0.99
P_GearB_fails = 0.005
P_GearB_works = 0.995
# Joint probability calculation
P_EngW_GearBW = P_Eng_works * P_GearB_works
P_EngF_GearBF = P_Eng_fails * P_GearB_fails
# Calculate result
P_fails_or_works = P_EngW_GearBW + P_EngF_GearBF
print(P_fails_or_works)
Deck of cards
# Ace probability
P_Ace = 4/52
# Not Ace probability
P_not_Ace = 1 — P_Ace
print(P_not_Ace)
# Figure probabilities
P_Hearts = 13/52
P_Diamonds = 13/52
# Probability of red calculation
P_Red = P_Hearts + P_Diamonds
print(P_Red)
# Figure probabilities
P_Jack = 4/52
P_Spade = 13/52
# Joint probability
P_Jack_n_Spade = 1/52
# Probability of Jack or spade
P_Jack_or_Spade = P_Jack + P_Spade — P_Jack_n_Spade
print(P_Jack_or_Spade)
# Figure probabilities
P_King = 4/52
P_Queen = 4/52
# Joint probability
P_King_n_Queen = 0
# Probability of King or Queen
P_King_or_Queen = P_King + P_Queen — P_King_n_Queen
print(P_King_or_Queen)
Delayed flights
# Needed quantities
On_time = 241
Total_departures = 276
# Probability calculation
P_On_time = On_time / Total_departures
print(P_On_time)
# Needed quantities
P_On_time = 241 / 276
# Probability calculation
P_Delayed = 1 — P_On_time
print(P_Delayed)
# Needed quantities
Delayed_on_Tuesday = 24
On_Tuesday = 138
# Probability calculation
P_Delayed_g_Tuesday = Delayed_on_Tuesday / On_Tuesday
print(P_Delayed_g_Tuesday)
# Needed quantities
Delayed_on_Friday = 11
On_Friday = 138
# Probability calculation
P_Delayed_g_Friday = Delayed_on_Friday / On_Friday
print(P_Delayed_g_Friday)
Contingency table
# Individual probabilities
P_Red = 26/52
P_Red_n_Ace = 2/52
# Conditional probability calculation
P_Ace_given_Red = P_Red_n_Ace / P_Red
print(P_Ace_given_Red)
# Individual probabilities
P_Ace = 4/52
P_Ace_n_Black = 2/52
# Conditional probability calculation
P_Black_given_Ace = P_Ace_n_Black / P_Ace
print(P_Black_given_Ace)
# Individual probabilities
P_Black = 26/52
P_Black_n_Non_ace = 24/52
# Conditional probability calculation
P_Non_ace_given_Black = P_Black_n_Non_ace / P_Black
print(P_Non_ace_given_Black)
# Individual probabilities
P_Non_ace = 48/52
P_Non_ace_n_Red = 24/52
# Conditional probability calculation
P_Red_given_Non_ace = P_Non_ace_n_Red / P_Non_ace
print(P_Red_given_Non_ace)
More cards
P_first_Jack = 4/52
P_Jack_given_Jack = 3/51
# Joint probability calculation
P_two_Jacks = P_first_Jack * P_Jack_given_Jack
print(P_two_Jacks)
# Needed probabilities
P_Spade = 13/52
P_Spade_n_Ace = 1/52
# Conditional probability calculation
P_Ace_given_Spade = P_Spade_n_Ace / P_Spade
print(P_Ace_given_Spade)
# Needed probabilities
P_Face_card = 12/52
P_Face_card_n_Queen = 4/52
# Conditional probability calculation
P_Queen_given_Face_card = P_Face_card_n_Queen / P_Face_card
print(P_Queen_given_Face_card)
Formula 1 engines
# Needed probabilities
P_A = 0.7
P_last5000_g_A = 0.99
P_B = 0.3
P_last5000_g_B = 0.95
# Total probability calculation
P_last_5000 = P_A * P_last5000_g_A + P_B * P_last5000_g_B
print(P_last_5000)
Voters
# Individual probabilities
P_X = 0.43
# Conditional probabilities
P_Support_g_X = 0.53
# Total probability calculation
P_X_n_Support = P_X * P_Support_g_X
print(P_X_n_Support)
# Individual probabilities
P_Z = 0.32
# Conditional probabilities
P_Support_g_Z = 0.32
P_NoSupport_g_Z = 1 — P_Support_g_Z
# Total probability calculation
P_Z_n_NoSupport = P_Z * P_NoSupport_g_Z
print(P_Z_n_NoSupport)
# Individual probabilities
P_X = 0.43
P_Y = 0.25
P_Z = 0.32
# Conditional probabilities
P_Support_g_X = 0.53
P_Support_g_Y = 0.67
P_Support_g_Z = 0.32
# Total probability calculation
P_Support = P_X * P_Support_g_X + P_Y * P_Support_g_Y + P_Z * P_Support_g_Z
print(P_Support)
Conditioning
In many situations we find events that depend on other events, and we are interested in calculating probabilities taking into consideration such relations.
On the other hand, many problems can be studied by classifying the elements in our sample space. It is easier to work with classifications that do not have elements in common — i.e., that do not overlap. Using this context, please answer the following question:
· Why is Bayes’ rule important?
Possible Answers
· It allows you to calculate conditional probabilities.
· It allows you to calculate conditional probabilities for events that can be partitions in nonoverlapping parts. — Ans
· It allows you to calculate joint probabilities.
· It was discovered by the Presbyterian minister Thomas Bayes.
Factories and parts
# Individual probabilities & conditional probabilities
P_V1 = 0.5
P_V2 = 0.25
P_V3 = 0.25
P_D_g_V1 = 0.01
P_D_g_V2 = 0.02
P_D_g_V3 = 0.03
# Probability of Damaged
P_Damaged = (P_V1 * P_D_g_V1) + (P_V2 * P_D_g_V2) + (P_V3 * P_D_g_V3)
# Bayes’ rule for P(V1|D)
P_V1_g_D = (P_V1 * P_D_g_V1) / P_Damaged
print(P_V1_g_D)
# Individual probabilities & conditional probabilities
P_V1 = 0.5
P_V2 = 0.25
P_V3 = 0.25
P_D_g_V1 = 0.01
P_D_g_V2 = 0.02
P_D_g_V3 = 0.03
# Probability of Damaged
P_Damaged = (P_V1 * P_D_g_V1) + (P_V2 * P_D_g_V2) + (P_V3 * P_D_g_V3)
# Bayes’ rule for P(V2|D)
P_V2_g_D = (P_V2 * P_D_g_V2) / P_Damaged
print(P_V2_g_D)
# Individual probabilities & conditional probabilities
P_V1 = 0.5
P_V2 = 0.25
P_V3 = 0.25
P_D_g_V1 = 0.01
P_D_g_V2 = 0.02
P_D_g_V3 = 0.03
# Probability of Damaged
P_Damaged = (P_V1 * P_D_g_V1) + (P_V2 * P_D_g_V2) + (P_V3 * P_D_g_V3)
# Bayes’ rule for P(V3|D)
P_V3_g_D = (P_V3 * P_D_g_V3) / P_Damaged
print(P_V3_g_D)
Swine flu blood test
# Probability of having Swine_flu
P_Swine_flu = 1./9000
# Probability of not having Swine_flu
P_no_Swine_flu = 1 — P_Swine_flu
# Probability of being positive given that you have Swine_flu
P_Positive_g_Swine_flu = 1
# Probability of being positive given that you do not have Swine_flu
P_Positive_g_no_Swine_flu = 0.01
# Probability of Positive
P_Positive = (P_Swine_flu * P_Positive_g_Swine_flu) + (P_no_Swine_flu * P_Positive_g_no_Swine_flu)
# Bayes’ rule for P(Swine_flu|Positive)
P_Swine_flu_g_Positive = (P_Swine_flu * P_Positive_g_Swine_flu) / P_Positive
print(P_Swine_flu_g_Positive)
# Individual probabilities & conditional probabilities
P_Swine_flu = 1./350
P_no_Swine_flu = 1 — P_Swine_flu
P_Positive_g_Swine_flu = 1
P_Positive_g_no_Swine_flu = 0.01
# Probability of Positive
P_Positive = (P_Swine_flu * P_Positive_g_Swine_flu) + (P_no_Swine_flu * P_Positive_g_no_Swine_flu)
# Bayes’ rule for P(Swine_flu|Positive)
P_Swine_flu_g_Positive = (P_Swine_flu * P_Positive_g_Swine_flu) / P_Positive
print(P_Swine_flu_g_Positive)
# Individual probabilities & conditional probabilities
P_Swine_flu = 1./350
P_no_Swine_flu = 1 — P_Swine_flu
P_Positive_g_Swine_flu = 1
P_Positive_g_no_Swine_flu = 0.02
# Probability of Positive
P_Positive = P_Swine_flu * P_Positive_g_Swine_flu + P_no_Swine_flu * P_Positive_g_no_Swine_flu
# Bayes’ rule for P(Swine_flu|Positive)
P_Swine_flu_g_Positive = (P_Swine_flu * P_Positive_g_Swine_flu) / P_Positive
print(P_Swine_flu_g_Positive)
Chapter-3
Range of values
Suppose the scores on a given academic test are normally distributed, with a mean of 65 and standard deviation of 10.
What would be the range of scores two standard deviations from the mean
Possible Answers
· 10 and 65
· 55 and 75
· 45 and 85 — Ans
· 35 and 95
Plotting normal distributions
# Import norm, matplotlib.pyplot, and seaborn
from scipy.stats import norm
import matplotlib.pyplot as plt
import seaborn as sns
# Create the sample using norm.rvs()
sample = norm.rvs(loc=3.15, scale=1.5, size=10000, random_state=13)
# Plot the sample
sns.distplot(sample)
plt.show()
Within three standard deviations
The heights of every employee in a company have been measured, and they are distributed normally with a mean of 168 cm and a standard deviation of 12 cm.
· What is the probability of getting a height within three standard deviations of the mean?
Possible Answers
· 68%
· 95%
· 99.7% — Ans
· 30%
Restaurant spending example
# Probability of spending $3 or less
spending = norm.cdf(3, loc=3.15, scale=1.5)
print(spending)
# Probability of spending more than $5
spending = norm.sf(5, loc=3.15, scale=1.5)
print(spending)
# Probability of spending more than $2.15 and $4.15 or less
spending_4 = norm.cdf(4.15, loc=3.15, scale=1.5)
spending_2 = norm.cdf(2.15, loc=3.15, scale=1.5)
print(spending_4 — spending_2)
# Probability of spending $2.15 or less or more than $4.15
spending_2 = norm.cdf(2.15, loc=3.15, scale=1.5)
spending_over_4 = norm.sf(4.15, loc=3.15, scale=1.5)
print(spending_2 + spending_over_4)
Smartphone battery example
# Probability that battery will last less than 3 hours
less_than_3h = norm.cdf(3, loc=5, scale=1.5)
print(less_than_3h)
# Probability that battery will last more than 3 hours
more_than_3h = norm.sf(3, loc=5, scale=1.5)
print(more_than_3h)
# Probability that battery will last between 5 and 7 hours
P_less_than_7h = norm.cdf(7, loc=5, scale=1.5)
P_less_than_5h = norm.cdf(5, loc=5, scale=1.5)
print(P_less_than_7h — P_less_than_5h)
Adults’ heights example
# Values one standard deviation from mean height for females
interval = norm.interval(0.68, loc=65, scale=3.5)
print(interval)
# Value where the tallest males fall with 0.01 probability
tallest = norm.ppf(0.99, loc=70, scale=4)
print(tallest)
# Probability of being taller than 73 inches for males and females
P_taller_male = norm.sf(73, loc=70, scale=4)
P_taller_female = norm.sf(73, loc=65, scale=3.5)
print(P_taller_male, P_taller_female)
# Probability of being shorter than 61 inches for males and females
P_shorter_male = norm.cdf(61, loc=70, scale=4)
P_shorter_female = norm.cdf(61, loc=65, scale=3.5)
print(P_shorter_male, P_shorter_female)
ATM example
# Import poisson from scipy.stats
from scipy.stats import poisson
# Probability of more than 1 customer
probability = poisson.sf(k=1, mu=1)
# Print the result
print(probability)
Highway accidents example
# Import the poisson object
from scipy.stats import poisson
# Probability of 5 accidents any day
P_five_accidents = poisson.pmf(k=5, mu=2)
# Print the result
print(P_five_accidents)
# Import the poisson object
from scipy.stats import poisson
# Probability of having 4 or 5 accidents on any day
P_less_than_6 = poisson.cdf(k=5, mu=2)
P_less_than_4 = poisson.cdf(k=3, mu=2)
# Print the result
print(P_less_than_6 — P_less_than_4)
# Import the poisson object
from scipy.stats import poisson
# Probability of more than 3 accidents any day
P_more_than_3 = poisson.sf(k=3, mu=2)
# Print the result
print(P_more_than_3)
# Import the poisson object
from scipy.stats import poisson
# Number of accidents with 0.75 probability
accidents = poisson.ppf(q=0.75, mu=2)
# Print the result
print(accidents)
Generating and plotting Poisson distributions
# Import poisson, matplotlib.pyplot, and seaborn
from scipy.stats import poisson
import matplotlib.pyplot as plt
import seaborn as sns
# Create the sample
sample = poisson.rvs(mu=2, size=10000, random_state=13)
# Plot the sample
sns.distplot(sample, kde=False)
plt.show()
Catching salmon example
# Getting a salmon on the third attempt
probability = geom.pmf(k=3, p=0.0333)
# Print the result
print(probability)
# Probability of getting a salmon in less than 5 attempts
probability = geom.cdf(k=4, p=0.0333)
# Print the result
print(probability)
# Probability of getting a salmon in less than 21 attempts
probability = geom.cdf(k=20, p=0.0333)
# Print the result
print(probability)
# Attempts for 0.9 probability of catching a salmon
attempts = geom.ppf(q=0.9, p=0.0333)
# Print the result
print(attempts)
Free throws example
# Import geom from scipy.stats
from scipy.stats import geom
# Probability of missing first and scoring on second throw
probability = geom.pmf(k=2, p=0.3)
# Print the result
print(probability)
Generating and plotting geometric distributions
# Import geom, matplotlib.pyplot, and seaborn
from scipy.stats import geom
import matplotlib.pyplot as plt
import seaborn as sns
# Create the sample
sample = geom.rvs(p=0.3, size=10000, random_state=13)
# Plot the sample
sns.distplot(sample, bins = np.linspace(0,20,21), kde=False)
plt.show()
Chapter-4
Generating a sample
# Import the binom object
from scipy.stats import binom
# Generate a sample of 250 newborn children
sample = binom.rvs(n=1, p=0.505, size=250, random_state=42)
# Show the sample values
print(sample)
Calculating the sample mean
# Print the sample mean of the first 10 samples
print(describe(sample[0:10]).mean)
# Print the sample mean of the first 50 samples
print(describe(sample[0:50]).mean)
# Print the sample mean of the first 250 samples
print(describe(sample[0:250]).mean)
Plotting the sample mean
# Calculate sample mean and store it on averages array
averages = []
for i in range(2, 251):
averages.append(describe(sample[0:i]).mean)
# Calculate sample mean and store it on averages array
averages = []
for i in range(2, 251):
averages.append(describe(sample[0:i]).mean)
# Add population mean line and sample mean plot
plt.axhline(binom.mean(n=1, p=0.505), color=’red’)
plt.plot(averages, ‘-’)
# Calculate sample mean and store it on averages array
averages = []
for i in range(2, 251):
averages.append(describe(sample[0:i]).mean)
# Add population mean line and sample mean plot
plt.axhline(binom.mean(n=1, p=0.505), color=’red’)
plt.plot(averages, ‘-’)
# Add legend
plt.legend((“Population mean”,”Sample mean”), loc=’upper right’)
plt.show()
Sample means
# Create list for sample means
sample_means = []
for _ in range(1500):
# Take 20 values from the population
sample = np.random.choice(population, 20)
# Create list for sample means
sample_means = []
for _ in range(1500):
# Take 20 values from the population
sample = np.random.choice(population, 20)
# Calculate the sample mean
sample_means.append(describe(sample).mean)
# Create list for sample means
sample_means = []
for _ in range(1500):
# Take 20 values from the population
sample = np.random.choice(population, 20)
# Calculate the sample mean
sample_means.append(describe(sample).mean)
# Plot the histogram
plt.hist(sample_means)
plt.xlabel(“Sample mean values”)
plt.ylabel(“Frequency”)
plt.show()
Question
Inspecting the plot, what is the distribution of the sample mean?
Possible Answers
· Same as the generated sample
· Binomial
· Normal –Ans
Sample means follow a normal distribution
# Generate the population
population = geom.rvs(p=0.5, size=1000)
# Create list for sample means
sample_means = []
for _ in range(3000):
# Take 20 values from the population
sample = np.random.choice(population, 20)
# Calculate the sample mean
sample_means.append(describe(sample).mean)
# Plot the histogram
plt.hist(sample_means)
plt.show()
# Generate the population
population = poisson.rvs(mu=2, size=1000)
# Create list for sample means
sample_means = []
for _ in range(1500):
# Take 20 values from the population
sample = np.random.choice(population, 20)
# Calculate the sample mean
sample_means.append(describe(sample).mean)
# Plot the histogram
plt.hist(sample_means)
plt.show()
Adding dice rolls
# Configure random generator
np.random.seed(42)
# Generate the sample
sample1 = roll_dice(2000)
# Plot the sample
plt.hist(sample1, bins=range(1, 8), width=0.9)
plt.show()
# Configure random generator
np.random.seed(42)
# Generate two samples of 2000 dice rolls
sample1 = roll_dice(2000)
sample2 = roll_dice(2000)
# Add the first two samples
sum_of_1_and_2 = np.add(sample1, sample2)
# Plot the sum
plt.hist(sum_of_1_and_2, bins=range(2, 14), width=0.9)
plt.show()
# Configure random generator
np.random.seed(42)
# Generate the samples
sample1 = roll_dice(2000)
sample2 = roll_dice(2000)
sample3 = roll_dice(2000)
# Add the first two samples
sum_of_1_and_2 = np.add(sample1, sample2)
# Add the first two with the third sample
sum_of_3_samples = np.add(sum_of_1_and_2, sample3)
# Plot the result
plt.hist(sum_of_3_samples, bins=range(3, 20), width=0.9)
plt.show()
Fitting a model
# Import the linregress() function
from scipy.stats import linregress
# Get the model parameters
slope, intercept, r_value, p_value, std_err = linregress(hours_of_study, scores)
# Print the linear model parameters
print(‘slope:’, slope)
print(‘intercept:’, intercept)
Predicting test scores
# Get the predicted test score for given hours of study
score = slope*10 + intercept
print(‘score:’, score)
# Get the predicted test score for given hours of study
score = slope*9 + intercept
print(‘score:’, score)
# Get the predicted test score for given hours of study
score = slope*12 + intercept
print(‘score:’, score)
Studying residuals
# Scatterplot of hours of study and test scores
plt.scatter(hours_of_study_A, test_scores_A)
# Plot of hours_of_study_values_A and predicted values
plt.plot(hours_of_study_values_A, model_A.predict(hours_of_study_values_A))
plt.title(“Model A”, fontsize=25)
plt.show()
# Calculate the residuals
residuals_A = model_A.predict(hours_of_study_A) — test_scores_A
# Make a scatterplot of residuals of model_A
plt.scatter(hours_of_study_A, residuals_A)
# Add reference line and title and show plot
plt.hlines(0, 0, 30, colors=’r’, linestyles=’ — ‘)
plt.title(“Residuals plot of Model A”, fontsize=25)
plt.show()
# Scatterplot of hours of study and test scores
plt.scatter(hours_of_study_B, test_scores_B)
# Plot of hours_of_study_values_B and predicted values
plt.plot(hours_of_study_values_B, model_B.predict(hours_of_study_values_B))
plt.title(“Model B”, fontsize=25)
plt.show()
# Calculate the residuals
residuals_B = model_B.predict(hours_of_study_B) — test_scores_B
# Make a scatterplot of residuals of model_B
plt.scatter(hours_of_study_B, residuals_B)
# Add reference line and title and show plot
plt.hlines(0, 0, 30, colors=’r’, linestyles=’ — ‘)
plt.title(“Residuals plot of Model B”, fontsize=25)
plt.show()
Fitting a logistic model
# Import LogisticRegression
from sklearn.linear_model import LogisticRegression
# sklearn logistic model
model = LogisticRegression(C=1e9)
model.fit(hours_of_study, outcomes)
# Get parameters
beta1 = model.coef_[0][0]
beta0 = model.intercept_[0]
# Print parameters
print(beta1, beta0)
Predicting if students will pass
# Specify values to predict
hours_of_study_test = [[10], [11], [12], [13], [14]]
# Pass values to predict
predicted_outcomes = model.predict(hours_of_study_test)
print(predicted_outcomes)
# Set value in array
value = np.asarray(11).reshape(-1,1)
# Probability of passing the test with 11 hours of study
print(“Probability of passing test “, model.predict_proba(value)[:,1])
Passing two tests
# Specify values to predict
hours_of_study_test_A = [[6], [7], [8], [9], [10]]
# Pass values to predict
predicted_outcomes_A = model_A.predict(hours_of_study_test_A)
print(predicted_outcomes_A)
# Specify values to predict
hours_of_study_test_B = [[3], [4], [5], [6]]
# Pass values to predict
predicted_outcomes_B = model_B.predict(hours_of_study_test_B)
print(predicted_outcomes_B)
# Set value in array
value_A = np.asarray(8.6).reshape(-1,1)
# Probability of passing test A with 8.6 hours of study
print(“The probability of passing test A with 8.6 hours of study is “, model_A.predict_proba(value_A)[:,1])
# Set value in array
value_B = np.asarray(4.7).reshape(-1,1)
# Probability of passing test B with 4.7 hours of study
print(“The probability of passing test B with 4.7 hours of study is “, model_B.predict_proba(value_B)[:,1])
# Print the hours required to have 0.5 probability on model_A
print(“Minimum hours of study for test A are “, -model_A.intercept_/model_A.coef_)
# Print the hours required to have 0.5 probability on model_B
print(“Minimum hours of study for test B are “, -model_B.intercept_/model_B.coef_)
# Probability calculation for each value of study_hours
prob_passing_A = model_A.predict_proba(study_hours_A.reshape(-1,1))[:,1]
prob_passing_B = model_B.predict_proba(study_hours_B.reshape(-1,1))[:,1]
# Calculate the probability of passing both tests
prob_passing_A_and_B = prob_passing_A * prob_passing_B
# Maximum probability value
max_prob = max(prob_passing_A_and_B)
# Position where we get the maximum value
max_position = np.where(prob_passing_A_and_B == max_prob)[0][0]
# Study hours for each test
print(“Study {:1.0f} hours for the first and {:1.0f} hours for the second test and you will pass both tests with {:01.2f} probability.”.format(study_hours_A[max_position], study_hours_B[max_position], max_prob))
▬▬▬▬▬▬ Connect with me ▬▬▬▬▬▬
Youtube Subscription ► https://bit.ly/2LENtS1
Facebook Page: ► https://www.facebook.com/EasyAWSLearn/
Blog: ► https://easyawslearn.blogspot.com/
Dev: ► https://dev.to/easyawslearn
Telegram Channel: ► https://t.me/devtul